Share
Back to blog
Top 10 Best AI Agents to Enhance Your Productivity in 2025
Contributor
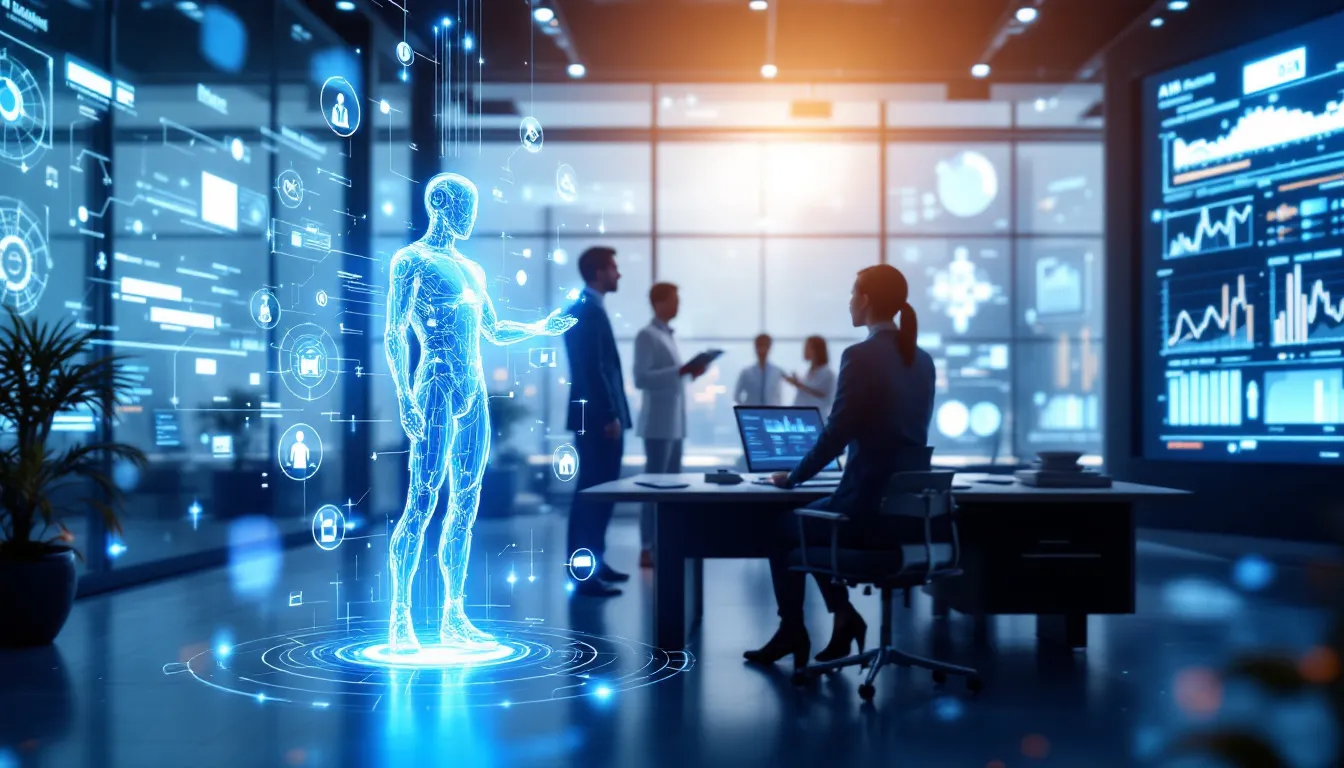
Top 10 Best AI Agents to Enhance Your Productivity in 2025
Introduction
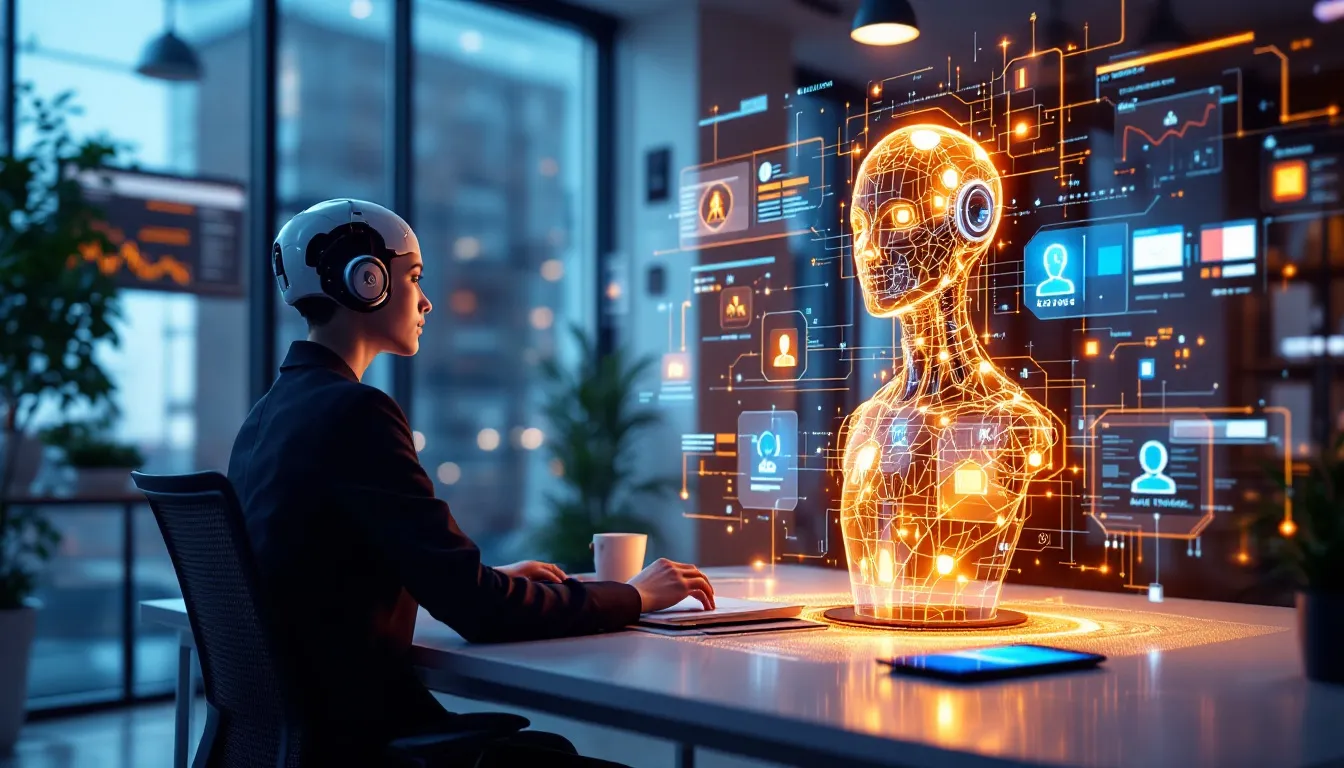
As AI agents become part of our daily lives, they’ve shifted from futuristic concepts to essential digital assistants, ready to complete tasks you design them to do. They can help you optimize time, streamline tasks, and enhance productivity.
AI agents have come a long way—from basic automation to advanced task management and decision-making. In 2025, they will be more intuitive and responsive, supporting diverse needs in project management, content creation, and even coding. These agents now handle everything from automating repetitive tasks to offering intelligent recommendations, making them invaluable for professionals, students, and creators alike.
This article covers the top 10 AI agents you need to know in 2025, each serving different use case. Whether you need help with task management, research, communication, or creative work, these tools are ready to make this year your most productive yet.
Introduction to AI Agents

AI agents are specialized software systems designed to autonomously perform specific tasks, often by analyzing data, making decisions, and learning from interactions. You can think of it as an AI assistant that not only answers questions but can use tools and perform tasks end-to-end. Unlike traditional software, which follows pre-defined instructions, AI agents use machine learning and natural language processing to adapt and respond dynamically based on the data they encounter. This flexibility allows AI agents to handle complex, evolving tasks more effectively than standard software, providing intelligent recommendations.
What is an AI Agent?

An AI agent is a software program designed to perform tasks autonomously or semi-autonomously on behalf of a user. It uses artificial intelligence to make decisions, complete actions, and continuously improve its capabilities. These agents can range from simple bots that automate routine tasks to advanced assistants that learn from data, adapt to user preferences, and carry out complex operations across various applications and platforms. Their functionality often combines machine learning, natural language processing, and decision-making algorithms, enabling them to respond intelligently to a user’s needs and instructions.
Distinction Between AI Agents and Traditional Software

The key difference between AI agents and traditional software lies in adaptability and autonomy. Traditional software executes fixed commands; it doesn’t change or improve its behavior without manual updates. In contrast, AI agents learn from data and refine their responses over time, enabling them to solve problems in new ways. This makes AI agents ideal for tasks that benefit from continuous learning and optimization, positioning them as essential tools in environments where quick adaptation is critical.
Types of AI agents

As Wordware team we encourage people to think of AI agents as of interns—if you can describe the standard operating procedure to a human being and they will be able to perform the task after couple of times you check their results, it means it's a perfect use case for an agent. It's important to remember that since AI agents now are mainly built on top of LLMs they perform best at tasks that include natural language processing.
AI agents can be categorized into several types, each with unique capabilities suited for different tasks. Understanding these distinctions can help businesses choose the right kind of agent for their specific needs. Here’s an overview of the five main types:
1. Reactive Agents
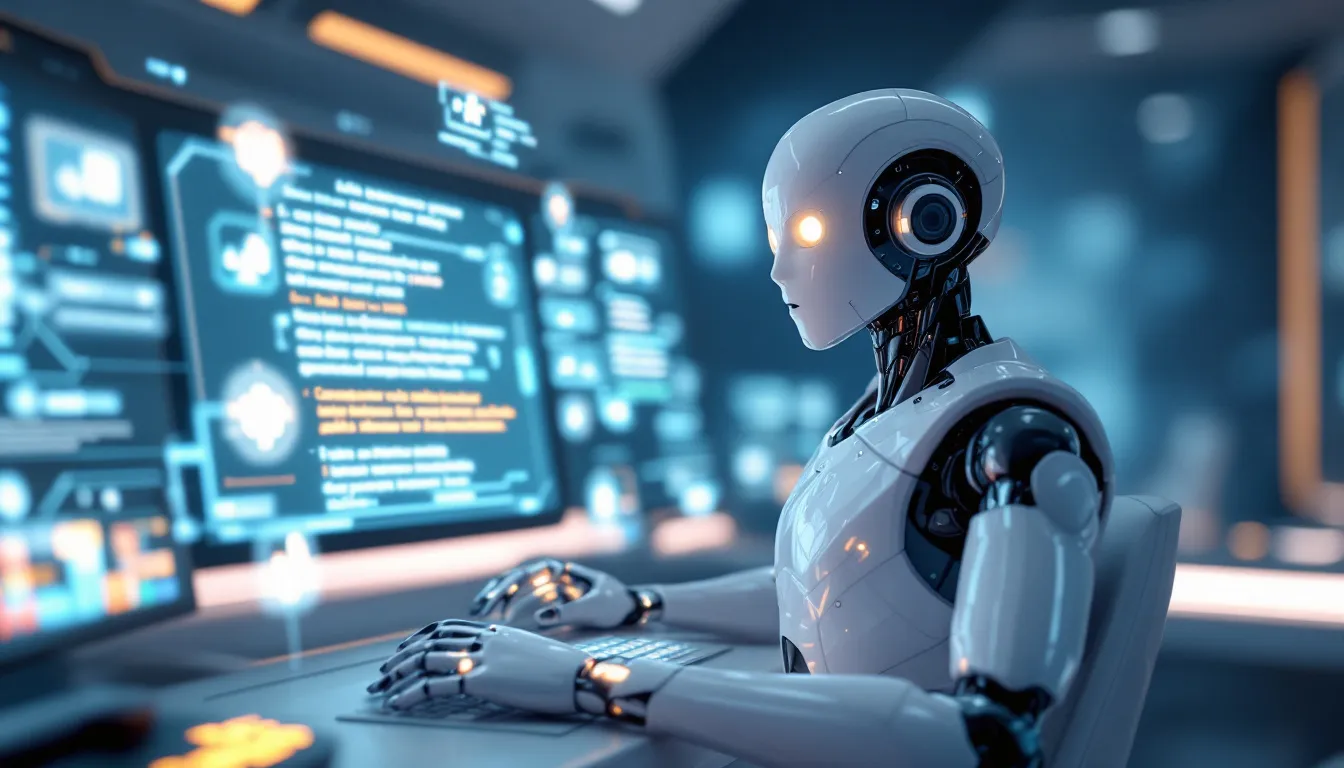
Reactive agents are the simplest form of AI, focusing on immediate responses based on current inputs without relying on past experiences. They don’t store information about previous actions, meaning they can’t adapt or learn over time. These agents are ideal for straightforward, rule-based tasks, like basic chatbots or systems that respond to specific commands with fixed outputs.
2. Deliberative Agents
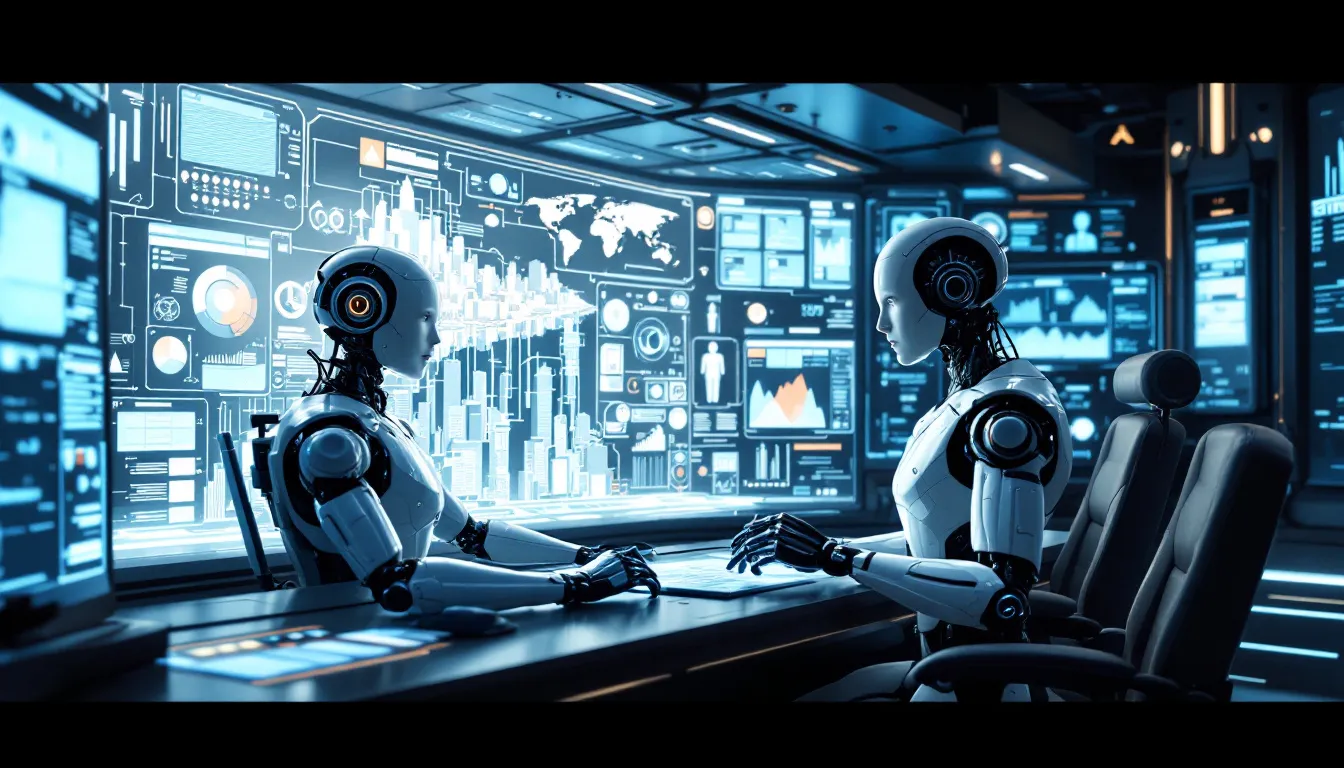
Deliberative agents, also known as model-based agents, operate by creating an internal model of the world around them. They can plan, reason, and make decisions based on a set of goals, allowing them to perform more complex tasks than reactive agents. This type is often used in applications where decision-making and goal-oriented behavior are essential, such as navigation systems or strategic planning tools.
3. Hybrid Agents
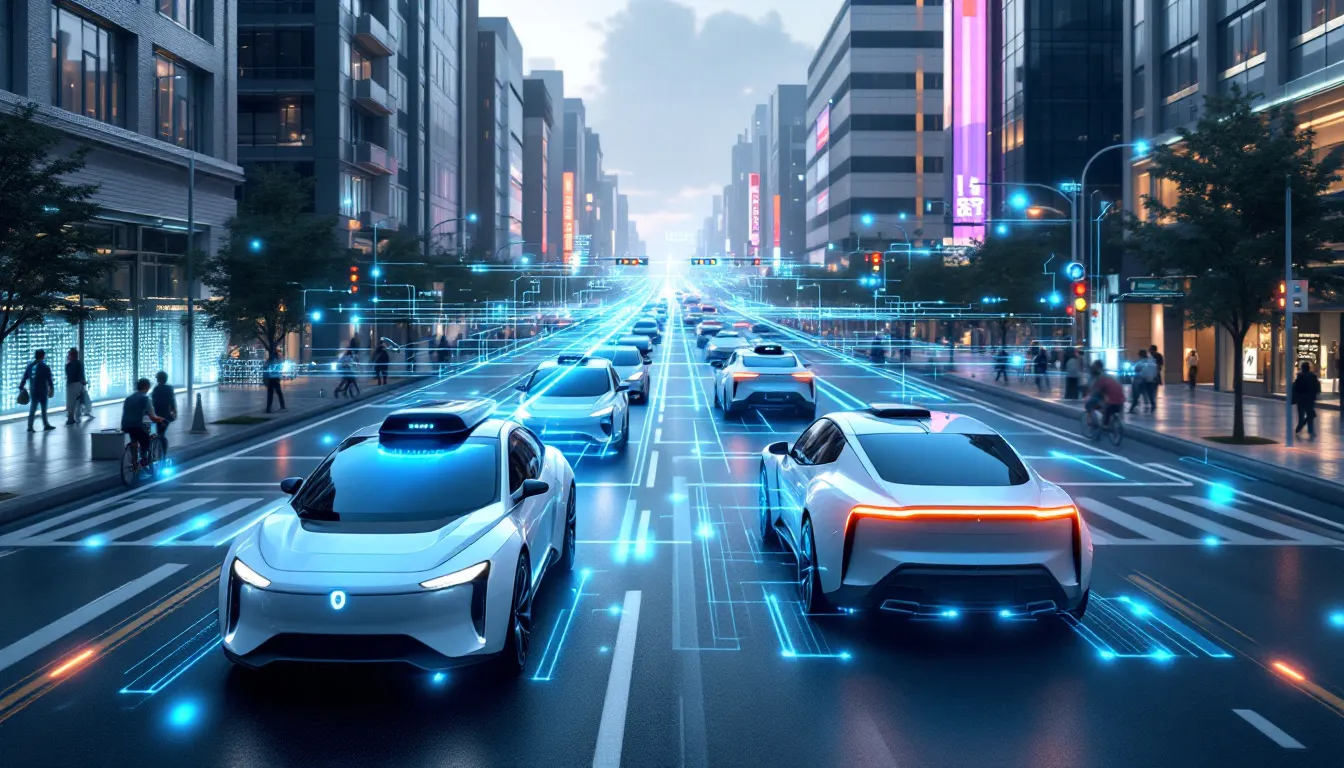
Hybrid agents combine reactive and deliberative approaches to balance immediate responses with goal-based decision-making. By merging these capabilities, hybrid agents can quickly adapt to changes while still working toward long-term objectives. They are widely used in situations requiring both flexibility and structured planning, like autonomous driving systems.
4. Learning Agents
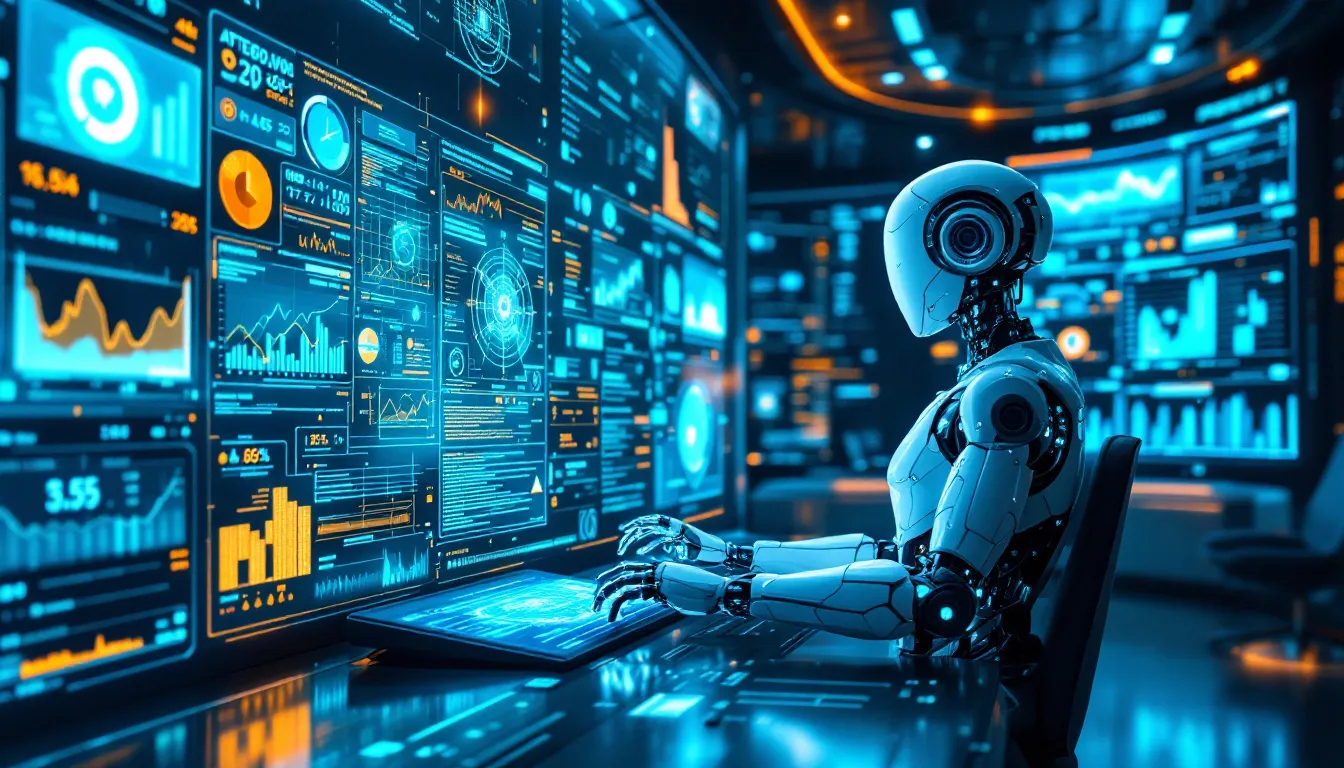
Learning agents have the ability to improve their performance over time by learning from interactions and feedback. Using machine learning, they adjust their behavior based on previous experiences, making them well-suited for tasks where adaptability is critical, such as recommendation engines or predictive analytics.
5. Autonomous Agents
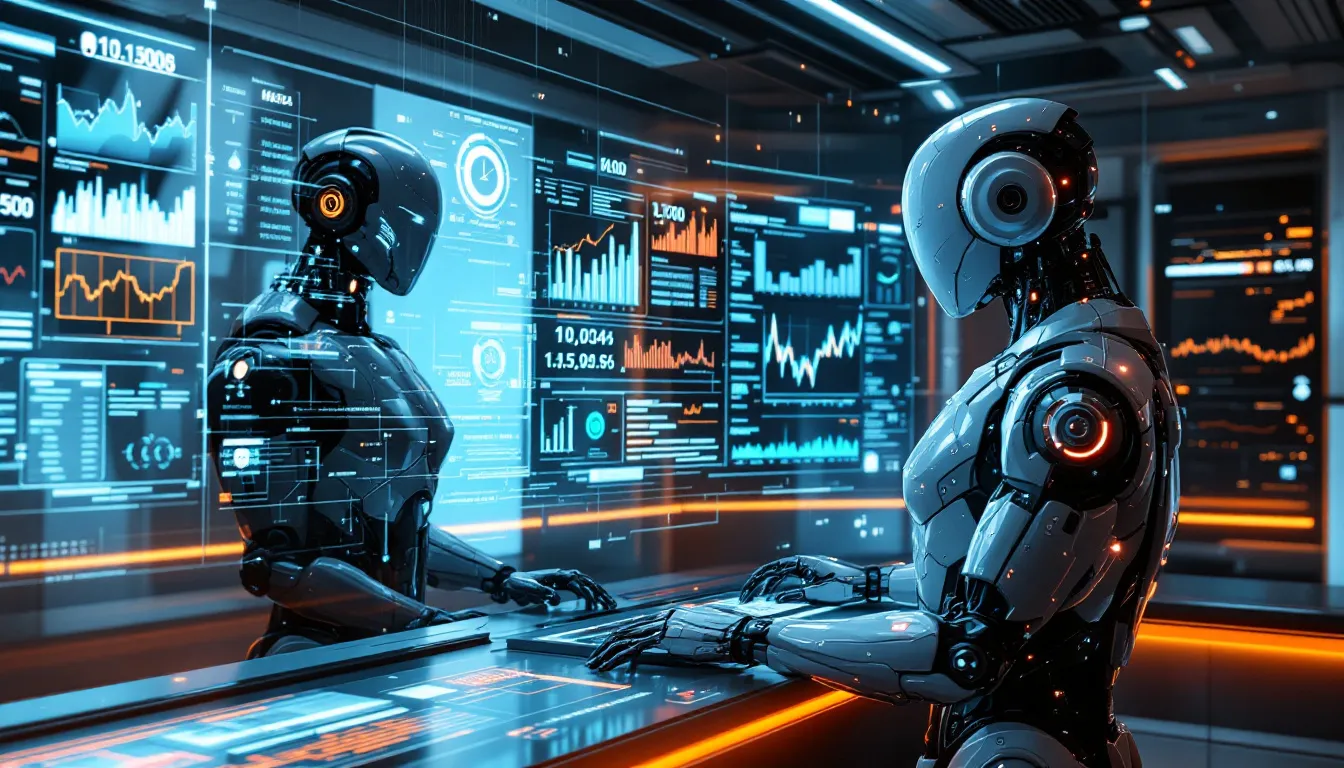
Autonomous AI agent operates independently, with minimal or no human intervention. They make complex decisions based on data, goals, and the environment, often using a combination of reactive, deliberative, and learning capabilities. Autonomous agents are used in high-stakes environments like financial trading, healthcare, and robotics, where adaptability and decision-making are essential.
What is the ideal agent in AI?

The ideal agent in AI should possess several key attributes:
Flexibility: Ability to adapt to different tasks across various domains.
Efficiency: Capable of processing large datasets quickly while minimizing errors.
User-Friendly Interface: Easy for users to interact with without extensive training.
We believe that in 2025 the best agents are the more narrow, hand crafted ones. Their goal is to follow the task step by step with no autonomy or decision making. That makes these agents the most reliable of all, and ideal for automating repetitive tasks. We could also distinguish autonomous agents and semi-autonomous ai agents. These ones can make decisions on their own and could be use for more complex tasks, where the process of execution isn't always is the same.
Characteristics of a Robust AI Agent
A robust AI agent should possess certain characteristics that enable it to perform complex tasks efficiently and effectively. These characteristics include:
Multimodal, Operational, and Sharable but also Separable
A robust AI agent should be able to process and integrate multiple types of data, such as text, images, and audio, to provide a comprehensive understanding of the task at hand. This multimodal capability enables the agent to operate in a variety of environments and contexts. For instance, an AI agent that can analyze both visual and textual data can offer more accurate insights and perform more complex tasks, such as identifying trends in social media posts or analyzing customer feedback from multiple sources.
The agent should also be operational, meaning it can perform tasks autonomously without human intervention. This requires the agent to have a high degree of autonomy, allowing it to make decisions and take actions based on its own reasoning and planning. Autonomous operation is crucial for tasks that require real-time responses or continuous monitoring, such as financial trading or healthcare monitoring systems.
Furthermore, a robust AI agent should be sharable but also separable. This means that the agent can be shared across multiple platforms and devices, but also be able to operate independently and make decisions without relying on other agents or systems. For example, in a multi-agent framework, each agent can perform its specialized task while still contributing to the overall objective. This flexibility ensures that the system remains resilient and efficient, even if one agent encounters an issue.
AI Agent Tools
What are AI agent tools?

AI agent tools are software platforms designed to create, manage, and deploy AI-driven agents that automate specific tasks, make decisions, and learn over time. These tools allow developers and non-technical users to build intelligent agents tailored for various use cases, from customer support to data analysis, with minimal programming. By handling complex tasks autonomously, AI agent tools enable businesses to streamline processes and enhance productivity without needing a deep AI background.
Key Features of AI Agent Tools
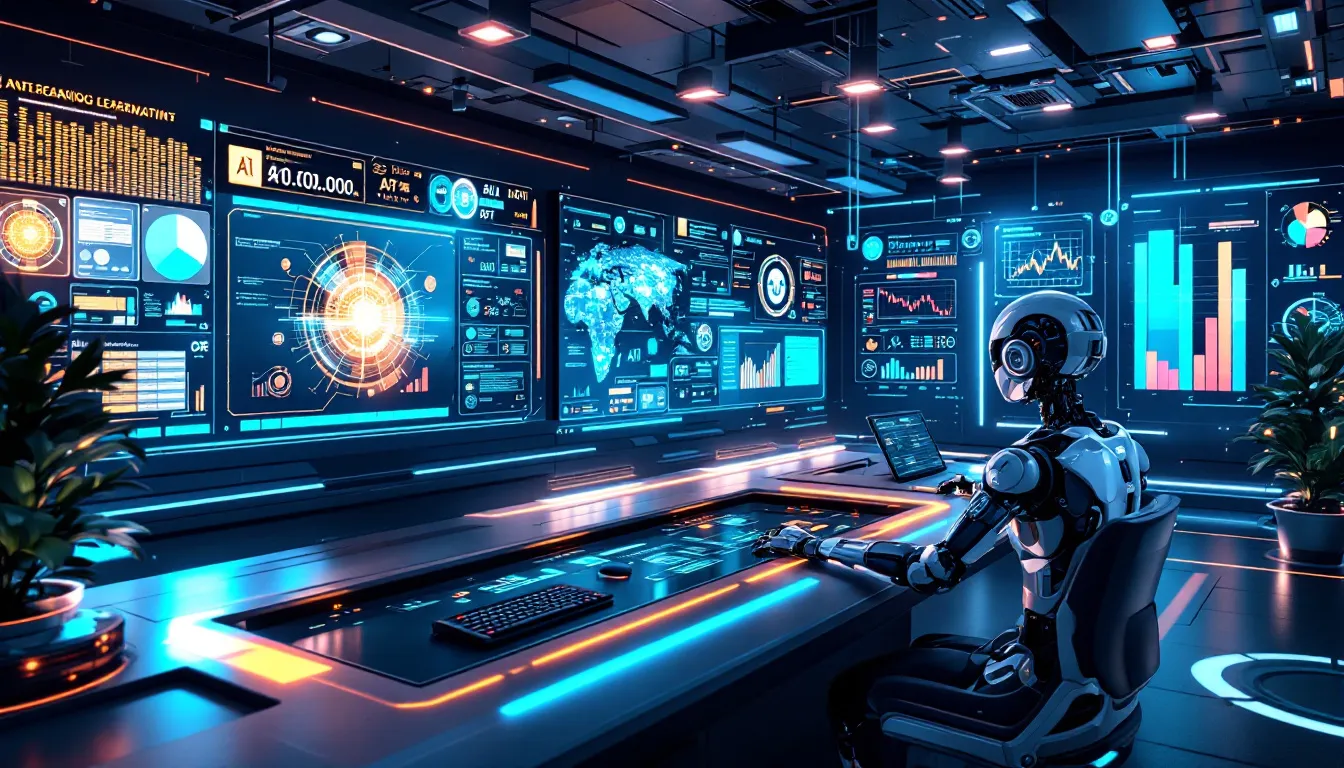
1. Learning
Learning capabilities in AI agent tools allow agents to improve their performance through data and feedback. With machine learning algorithms, agents adapt over time, refining their actions based on previous interactions. This is crucial in applications where agents must provide better recommendations, adapt to user behavior, or make increasingly accurate predictions.
2. Automation
AI agent tools often come with automation features that help streamline repetitive or high-volume tasks. By integrating with other software systems and APIs, these agents can carry out workflows across different applications without human intervention. This reduces manual effort and frees up resources for more strategic activities, whether in customer support, data entry, or business reporting.
3. Decision-Making
Decision-making functionality enables agents to analyze information, evaluate options, and take actions based on defined goals and real-time data. AI agents with decision-making capabilities can operate in dynamic environments, selecting the best course of action from multiple possibilities. This is essential for applications like financial trading, supply chain management, and autonomous systems that require real-time responsiveness and judgment.
AI agent tools, with their learning, automation, and decision-making abilities, offer robust solutions for businesses aiming to automate complex tasks and achieve greater efficiency. These tools support a wide range of applications, making them a key part of the future of AI-driven operations.
Best AI Agents in 2025

In 2025, AI agents are playing an even more significant role across industries, helping professionals automate repetitive tasks, enhance productivity, and unlock new efficiencies. Here are the top 10 AI agents with unique features and use cases that are leading the way.
Top 10 AI Agents to Maximize Productivity and Efficiency
1. Research Agent
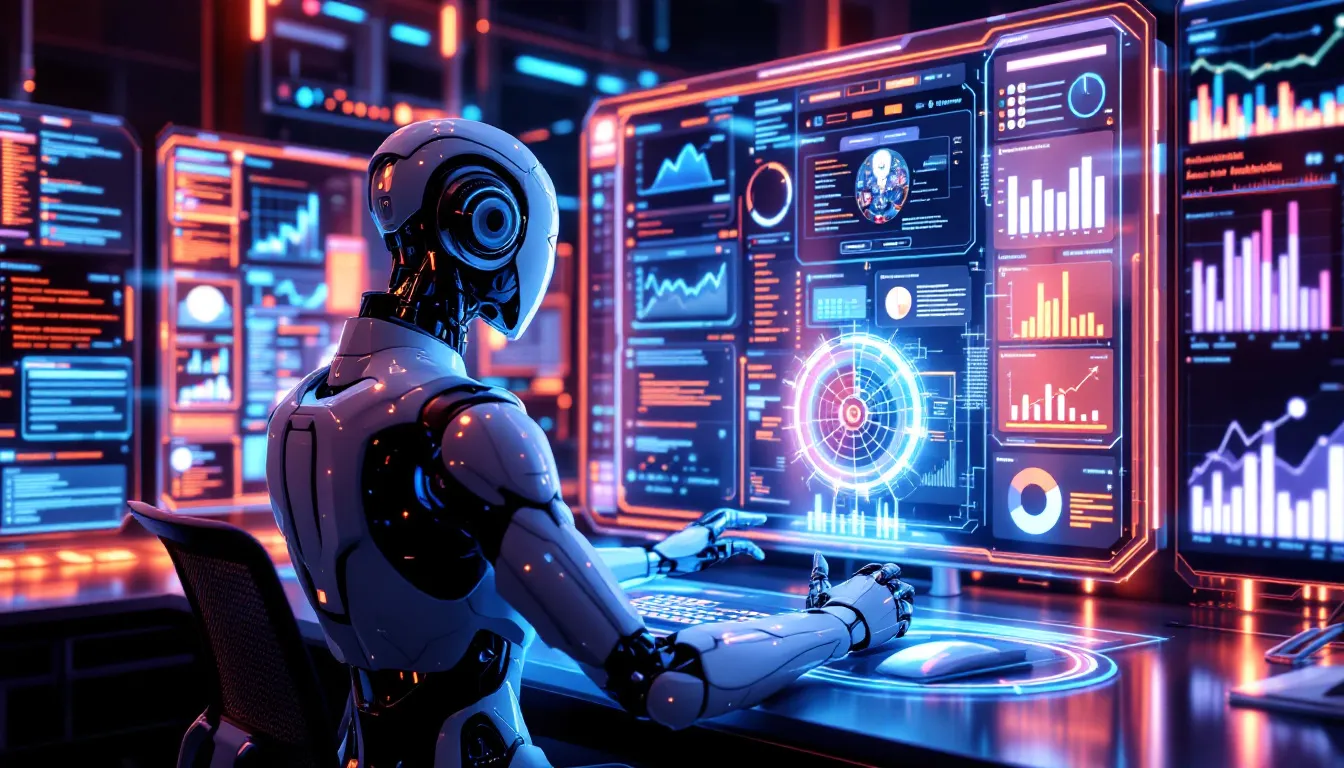
Features: Information gathering, summarization, citation management
Use Cases: Quickly collect and organize relevant data for projects, generate concise summaries, and manage references.
Research agents are invaluable for analysts, students, and professionals who need fast, accurate insights. These agents scan databases, extract relevant information, and compile summaries, speeding up the research process considerably.
For research-related tasks you can use Exa AI. They provide search engine that returns most relevant results for your query. If you want to see an agent that uses various sources to search for information, here you can use this free AI research agent - link.
2. Coding Agent

Features: Code generation, debugging assistance, syntax correction
Use Cases: Accelerate software development by generating clean code, troubleshooting errors, and providing recommendations.
Coding agents are ideal for developers looking to streamline their workflow. They can auto-generate code snippets based on requirements, spot potential bugs, and recommend improvements, making software development faster and more efficient.
A great example of coding agent is Cursor, which let's you prompt AI with natural language to code with and for you. If you can see how such agent might work, you can try for free this agent that will code the entire side-scroller game for you - link.
3. Copywriting Agent
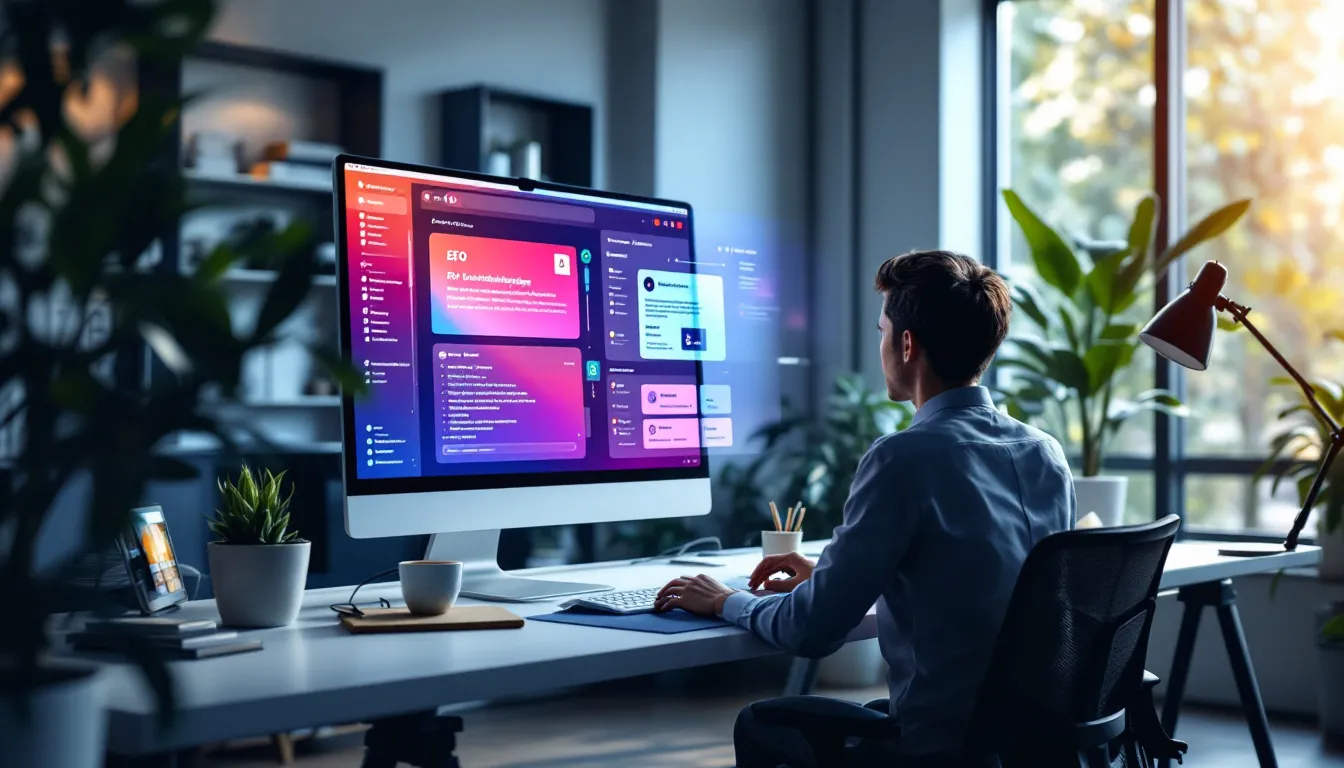
Features: Content generation, tone adjustment, SEO optimization
Use Cases: Produce high-quality marketing content, blog posts, and product descriptions tailored for specific audiences.
Copywriting agents are popular in marketing and content creation, as they can craft engaging, SEO-optimized content that matches brand tone and voice, saving hours on writing and editing. Try this free LinkedIn post writing AI agent to see it in action - link.
4. Learning Agent
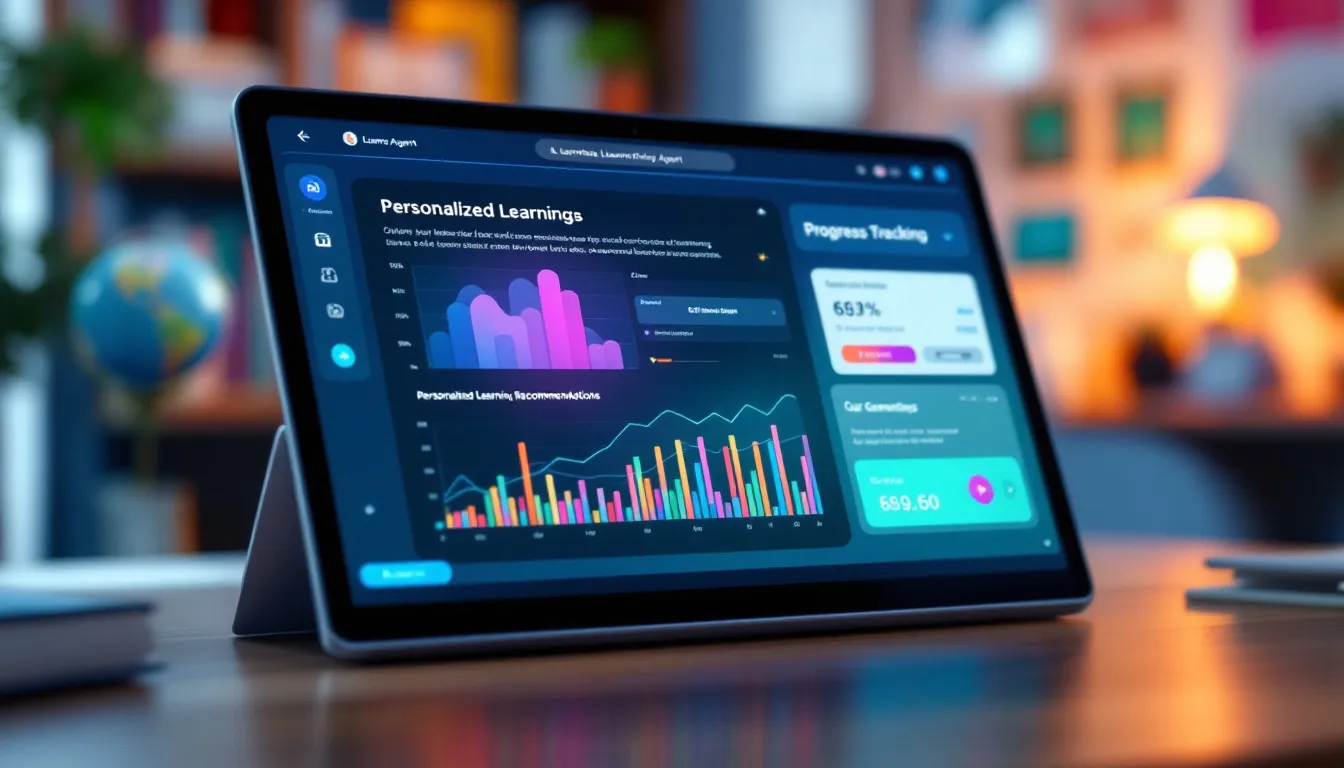
Features: Personalized learning recommendations, progress tracking, quiz generation
Use Cases: Customize learning experiences for students, employees, or personal development.
Learning agents can adapt educational content to individual learning styles and pace, providing quizzes, explanations, and study recommendations to boost knowledge retention and engagement. If you have something you'd like to learn, this free teaching AI agent can help you - link.
5. Legal Agent
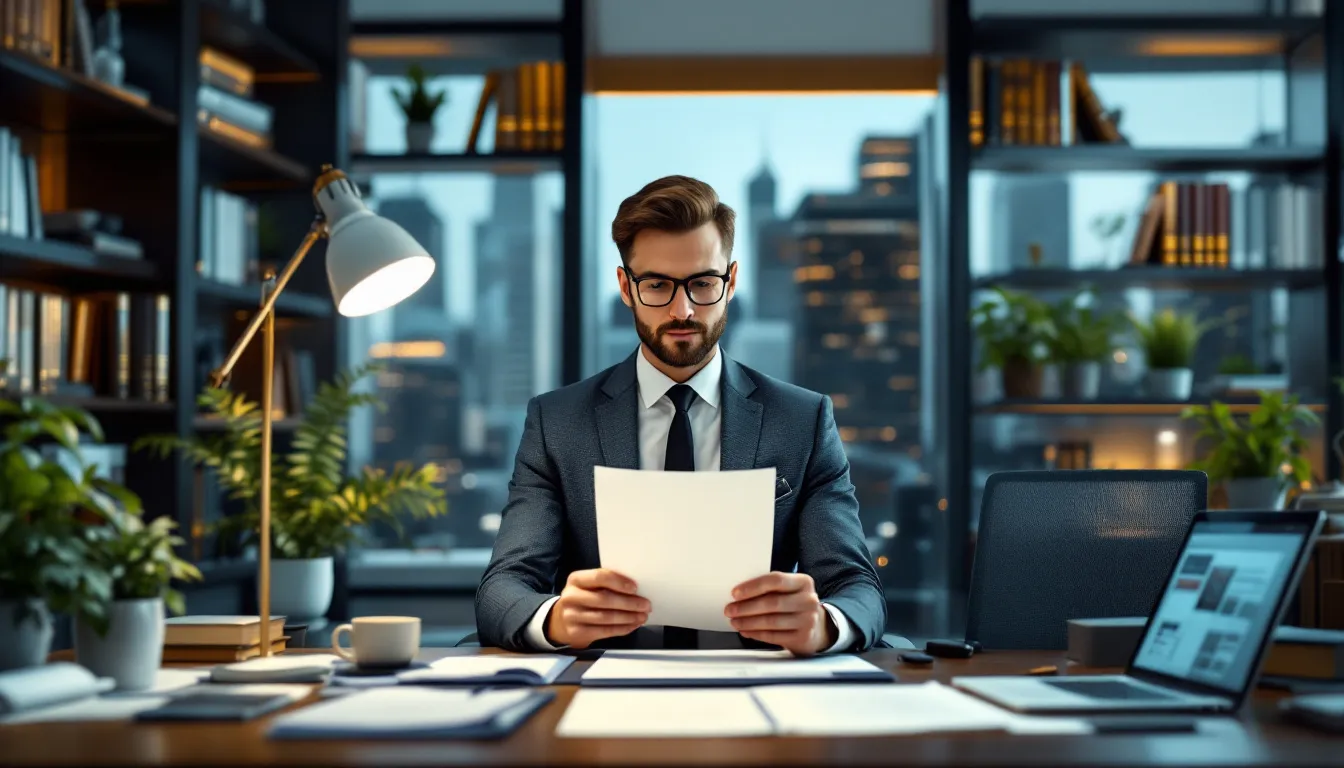
Features: Contract analysis, legal research, compliance checks
Use Cases: Draft and review contracts, perform legal research, and ensure documents meet compliance requirements.
Legal agents support law professionals by analyzing contracts, identifying potential risks, and staying updated on compliance regulations, significantly cutting down on manual legal review.
Harvey is one of the examples of company building agents in legal space. You can head here - link - to see example of free legal AI agent.
6. Job Interview Prep Agent

Features: Question generation, role-specific interview coaching, mock interview practice
Use Cases: Prepare candidates for interviews with personalized practice questions and feedback.
This agent is particularly useful for job seekers aiming to improve their interview performance. It provides tailored questions, coaches for specific job roles, and simulates interview scenarios for practice. If you want to use free AI for job interview preparation or you're busy professional who needs to prepare questions for candidate, see it in action here - link.
7. Data Analysis Agent
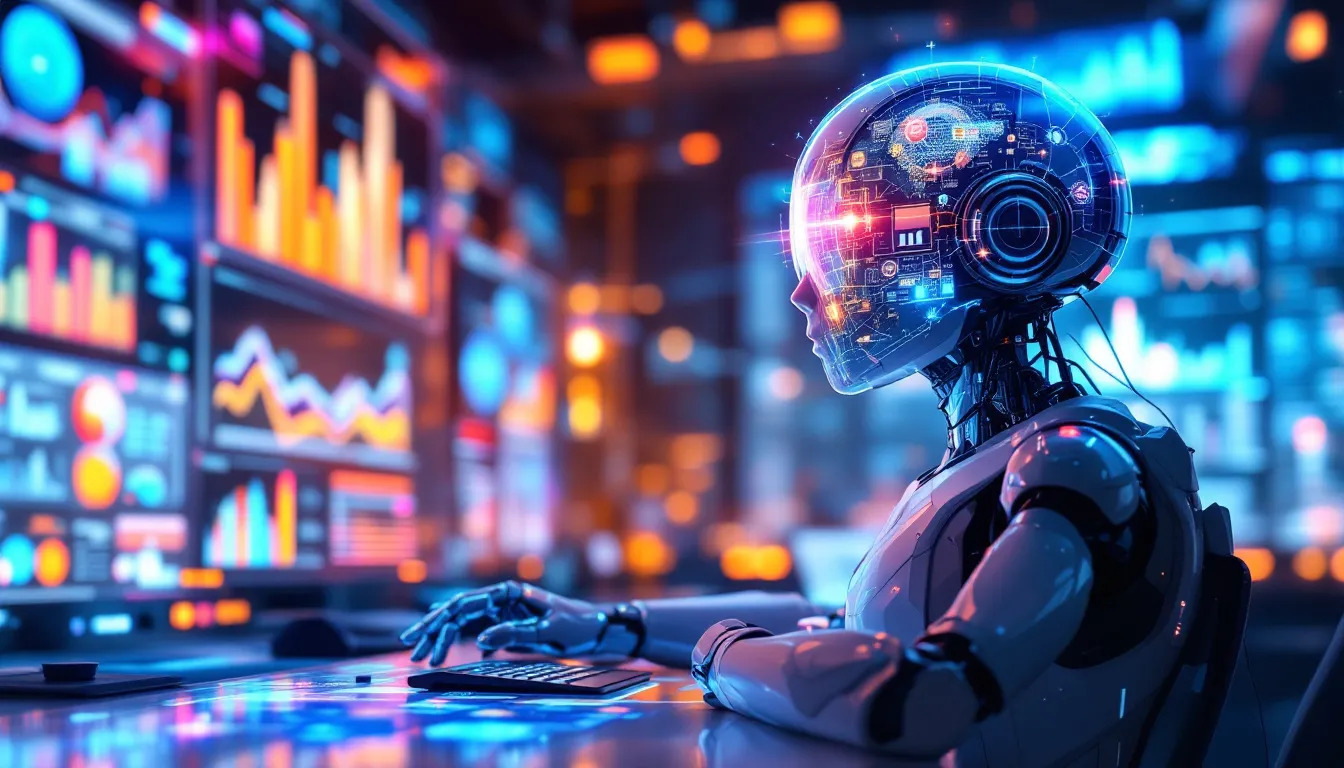
Features: Data cleaning, trend identification, visualization
Use Cases: Automate data preparation, identify patterns, and create visualizations for better decision-making.
Data analysis agents are valuable for teams handling large datasets, enabling them to extract data insights quickly without extensive data science expertise. These agents can process raw data, spot trends, and create charts and reports. An example could be Julius AI.
8. Customer Support Agent

Features: Multi-channel support, natural language processing, FAQ handling
Use Cases: Provide 24/7 support, answer customer inquiries, and resolve common issues.
Customer support agents use natural language processing to engage with customers across multiple platforms, answering FAQs and resolving issues autonomously, thereby improving customer satisfaction. If you want to see how you can include free AI agent for customer support that will enable them chatting with your website, head here - link.
9. Financial Planning Agent
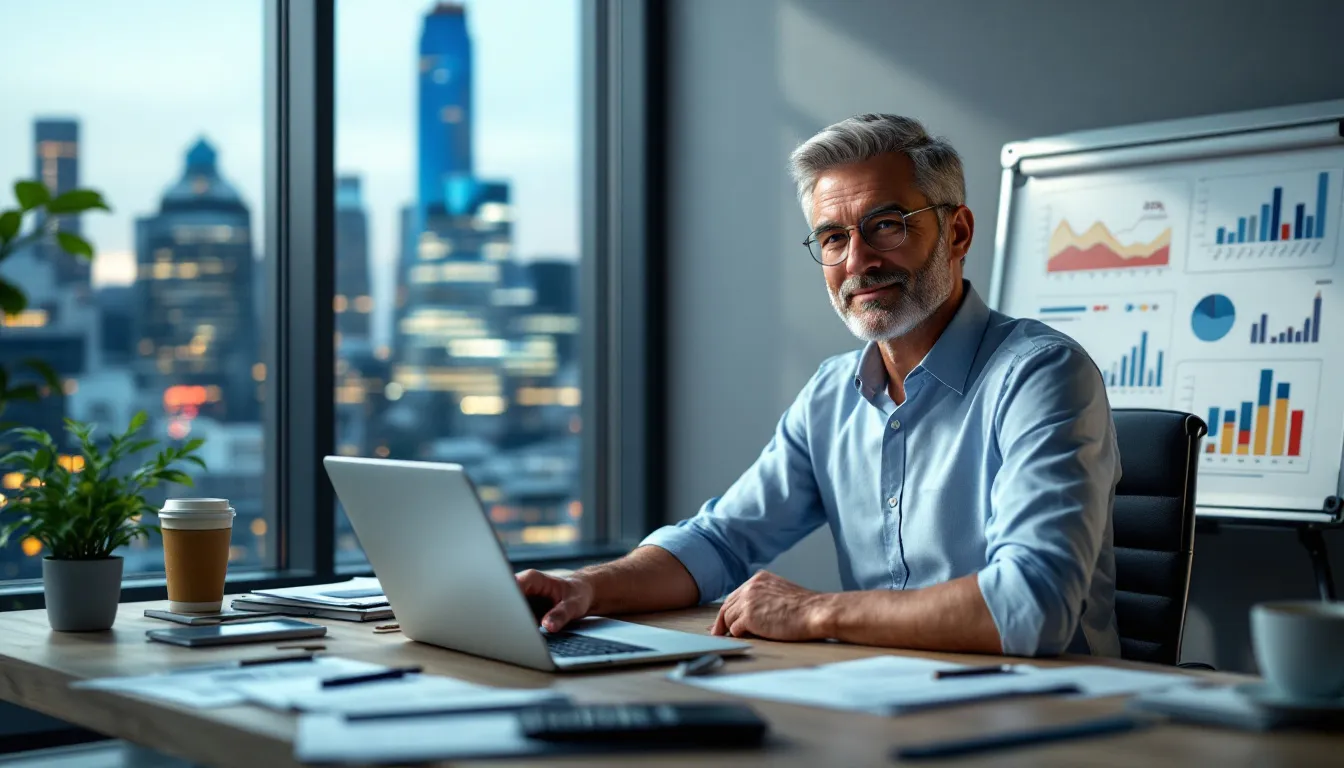
Features: Budget tracking, expense forecasting, investment analysis
Use Cases: Help individuals or businesses manage finances, create budgets, and analyze investments.
Financial planning agents support users in maintaining financial health by providing budgeting tips, tracking expenses, and recommending investment strategies, perfect for both personal finance and corporate budgeting. Want to use financial statement analysis AI for free? We've built that for you - link.
10. Creative Design Agent
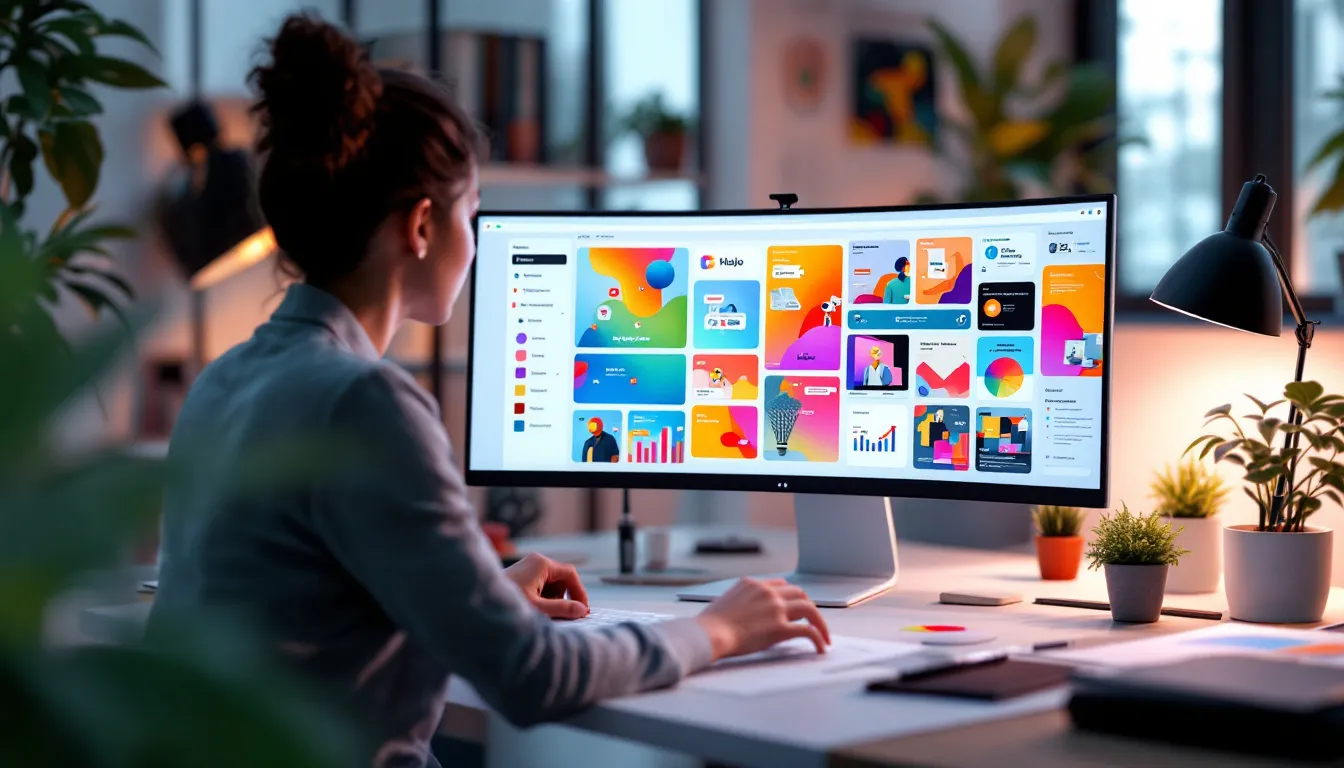
Features: Graphic design generation, layout optimization, brand style adherence
Use Cases: Generate on-brand visual content for marketing, social media, or branding materials.
Creative design agents assist designers and marketers by generating high-quality visuals that match brand guidelines. They can create layouts, optimize designs for various formats, and streamline content creation for digital campaigns.
Each of these AI agents leverages specialized features to address unique productivity needs, from research and coding to creative design and financial planning. They are becoming essential tools across sectors, helping users maximize efficiency, reduce manual effort, and focus on higher-level tasks.
Key Features and Use Cases of AI Agents
AI agents in 2025 come with a variety of advanced features that make them valuable across different industries. Here’s a look at some of the core capabilities these agents offer and how they are applied in real-world scenarios.
Key Features

1. Automation of Repetitive Tasks
AI agents are designed to handle repetitive, time-consuming tasks, such as data entry, document processing, or basic customer interactions. By automating these tasks, they free up users to focus on more strategic work.
2. Natural Language Processing (NLP)
Many AI agents are equipped with NLP to understand and generate human-like text. This enables them to respond to customer inquiries, draft emails, and create content that matches a specific tone or style, making them useful for roles in customer support and content creation.
3. Learning and Adaptation
Advanced AI agents are capable of learning from user feedback and adapting to specific requirements over time. This allows them to become more effective at tasks, like refining product recommendations or personalizing learning experiences.
4. Data Analysis and Insight Generation
AI agents with analytical features can process vast amounts of data, identifying patterns and trends that would take humans hours to spot. These agents are invaluable for roles in research, finance, and operations, where decision-making relies heavily on accurate data insights.
5. Integration with Other Tools and Platforms
Modern AI agents are designed to integrate seamlessly with other business software, like CRMs, project management platforms, and cloud storage solutions. This interoperability makes it easier to incorporate AI agents into existing workflows.
Use Cases

1. Customer Support
AI agents handle basic customer inquiries, resolving issues without human intervention and providing quick responses 24/7. This helps companies improve service response times and reduce the load on human agents.
2. Content Creation and Copywriting
Agents designed for copywriting can generate blog posts, product descriptions, or social media content tailored to specific audiences. They save marketers and content creators valuable time while ensuring that content remains engaging and on-brand.
3. Data Analysis and Reporting
Data analysis agents process complex datasets, produce visual reports, and highlight key trends. They are widely used in sectors like finance, healthcare, and market research, where data-driven insights are crucial for informed decisions.
4. Research and Document Summarization
Research agents scan large volumes of documents to extract relevant information and summarize findings. This feature benefits professionals in fields like law, academia, and consulting, where quick access to key insights is essential.
5. Project Management and Workflow Automation
AI agents streamline workflows by automating task assignments, tracking deadlines, and managing project resources. Teams can rely on these agents to keep projects on schedule and reduce administrative overhead.
By leveraging these key features, AI agents empower users to improve efficiency, reduce human error, and focus on high-impact work. These tools are transforming how people work, making complex tasks accessible and manageable even without specialized skills.
Which is the most powerful AI agent?
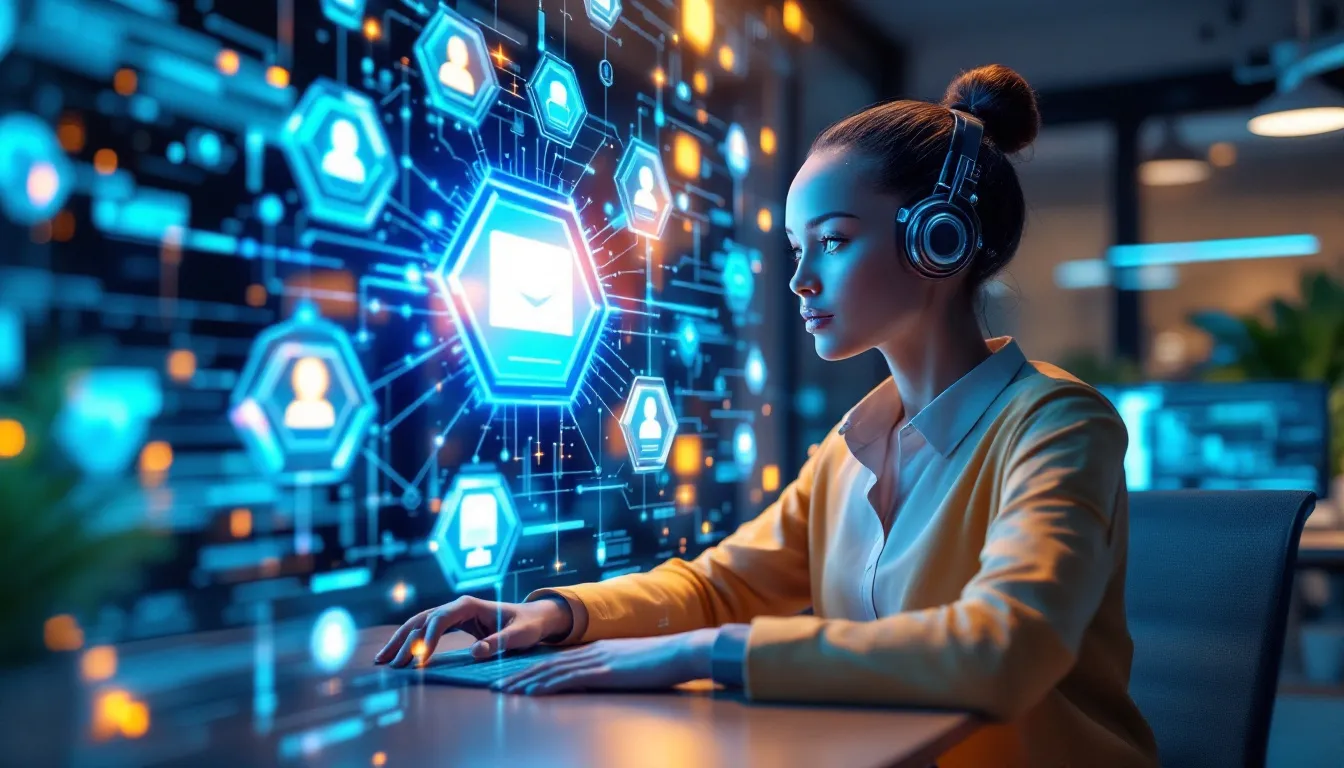
Determining the "most powerful" AI agent can be subjective as it depends on the criteria used for evaluation (e.g., versatility, performance). It's important to understand that AI agents perform best on tasks based on natural language processing or task requiring step-by-step reasoning.
Most powerful AI agents will be company's own agents. They should have ability to use tools, eg. gather information autonomously from the internet. By creating custom agent, providing it with ability to connect with other agents with simple agent integration, businesses can make sure they connect agents that will be powerful and perform tasks to standard required by the company.
Reasoning and Planning in AI Agents
Reasoning and planning are critical components of AI agents, enabling them to make decisions and take actions to achieve their goals. AI agents use various reasoning and planning techniques to enhance productivity and efficiency.
How AI Agents Use Reasoning and Planning to Enhance Productivity
AI agents use reasoning and planning to analyze complex tasks and break them down into smaller, manageable components. This enables the agent to identify the most efficient and effective way to complete the task, taking into account various factors such as time, resources, and constraints. For example, an AI agent tasked with project management can divide the project into individual tasks, assign priorities, and allocate resources accordingly.
The agent then uses planning techniques to schedule and execute the tasks, ensuring that the goals are achieved in a timely and efficient manner. This may involve coordinating with other agents or systems, allocating resources, and adapting to changing circumstances. For instance, in a multi-agent system, one agent might handle data collection while another focuses on data analysis, working together to complete the overall task more efficiently.
By using reasoning and planning, AI agents can enhance productivity by:
- Automating routine tasks and freeing up human resources for more complex and creative tasks.
- Optimizing workflows and processes to reduce waste and improve efficiency.
- Providing real-time insights and recommendations to support decision-making.
- Enabling autonomous operation and reducing the need for human intervention.
Overall, the characteristics of a robust AI agent, including multimodal, operational, and sharable but also separable capabilities, combined with advanced reasoning and planning techniques, enable AI agents to perform complex tasks efficiently and effectively, enhancing productivity and efficiency in a wide range of applications.
Autonomous AI Agents

Autonomous AI agents represent the next frontier in artificial intelligence, designed to operate with minimal human intervention. These agents perform complex tasks independently by perceiving their environment, making decisions, and taking actions based on pre-programmed goals or learned behaviors. Autonomous agents are especially valuable in dynamic or high-stakes environments where real-time, adaptive responses are critical.
What are autonomous agents in AI?
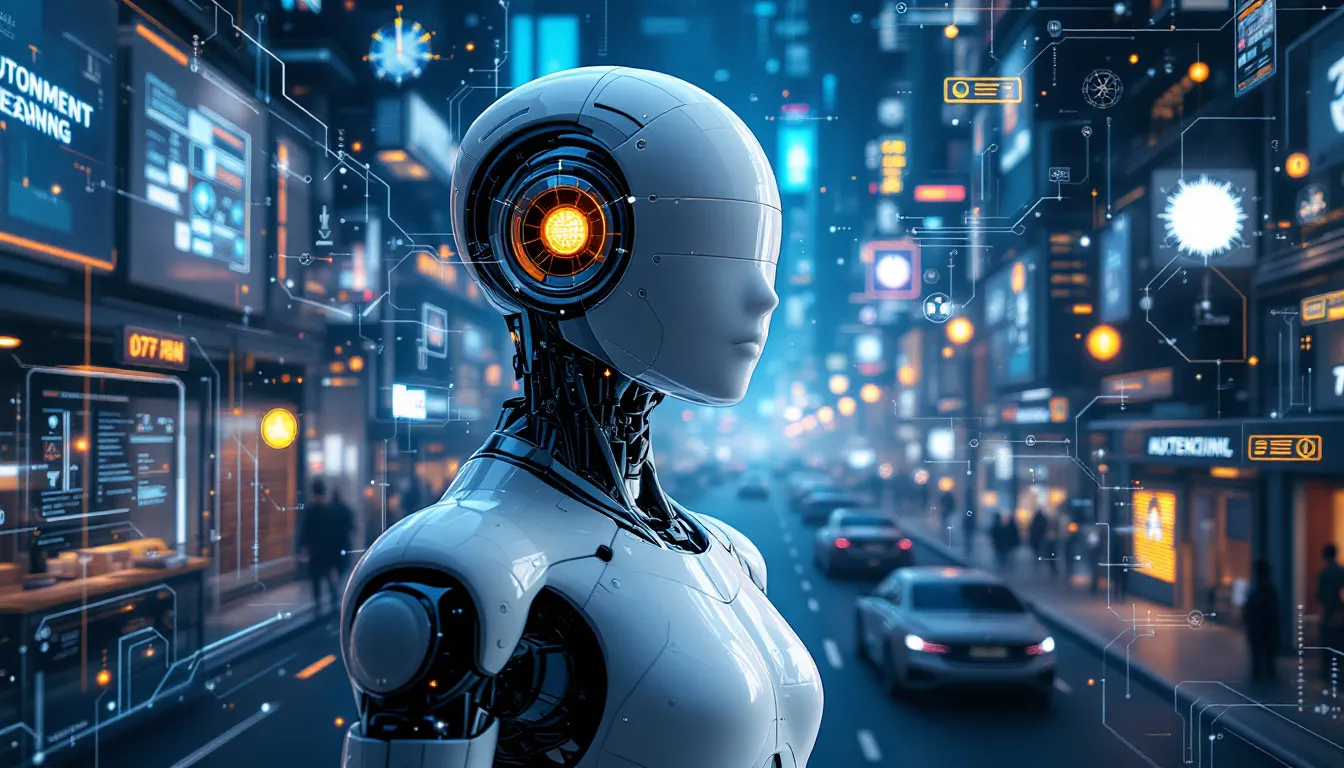
Autonomous agents are a subset of AI agents capable of operating independently without human intervention. They can perceive their environment through sensors or data inputs and take actions based on programmed objectives or learned experiences.
Characteristics and Benefits of Autonomous Agents
1. Self-Directed Decision-Making
Autonomous agents can analyze situations and choose the best course of action without needing constant oversight. They are designed to achieve specific objectives efficiently. They rely on algorithms that allow them to weigh options, consider potential outcomes, and make decisions aligned with their objectives. This self-direction is essential in fast-paced scenarios where immediate responses are necessary.
2. Continuous Learning and Adaptation
Many autonomous agents are designed to learn from interactions with their environment to improve decision-making. They learn from their experiences, becoming more effective over time and employ techniques like reinforcement learning to improve their decision-making skills and adapt to new challenges. This continuous learning allows them to remain effective as conditions evolve, whether in manufacturing, logistics, or personal assistance.
3. Context-Aware Operation
Autonomous agents excel at understanding and responding to contextual information. By analyzing data from various sources—sensors, GPS, or user input—they can adjust their actions to align with situational needs. This context awareness is invaluable in applications that demand real-time responses, such as self-driving cars or automated customer support systems.
4. High Scalability
Autonomous agents can operate at scale, managing large numbers of tasks or interactions without human intervention. This capability is particularly beneficial in industries where scalability and consistency are crucial, like customer service and data processing.
Examples of Autonomous Agents in Real-World Applications

1. Self-Driving Vehicles
Autonomous cars, such as those developed by companies like Tesla and Waymo, use an array of sensors, machine learning, and computer vision to navigate roads safely. These agents make real-time decisions on steering, speed, and route adjustments, reducing the need for a human driver while improving road safety and accessibility.
2. Warehouse Robotics
Autonomous robots in fulfillment centers, like those used by Amazon, handle tasks such as sorting, picking, and packing items. They operate independently, optimizing routes and workflows to increase efficiency and reduce operational costs, especially during peak periods.
3. Customer Service Chatbots
Autonomous customer service agents handle customer inquiries without human support, often responding with high accuracy. Equipped with NLP and self-learning capabilities, they adapt to common issues and user preferences over time, improving customer satisfaction and reducing service response times.
4. Healthcare Monitoring Systems
Autonomous healthcare agents monitor patients’ vital signs and alert medical personnel in emergencies. They operate in real-time, tracking data trends and recognizing early signs of health deterioration, particularly in elderly care or remote monitoring scenarios.
5. Financial Market Analysis and Trading
Autonomous trading bots analyze market data, execute trades, and adjust investment strategies autonomously. These agents can react instantly to market changes, leveraging data insights to make faster, more accurate decisions than human traders in high-frequency trading.
Autonomous agents are becoming critical assets across industries, enabling businesses to operate more efficiently and respond quickly to complex challenges. Their ability to function independently while learning and adapting ensures they can deliver consistent results, especially in situations where human intervention is limited or costly.
Frameworks for Building AI Agents
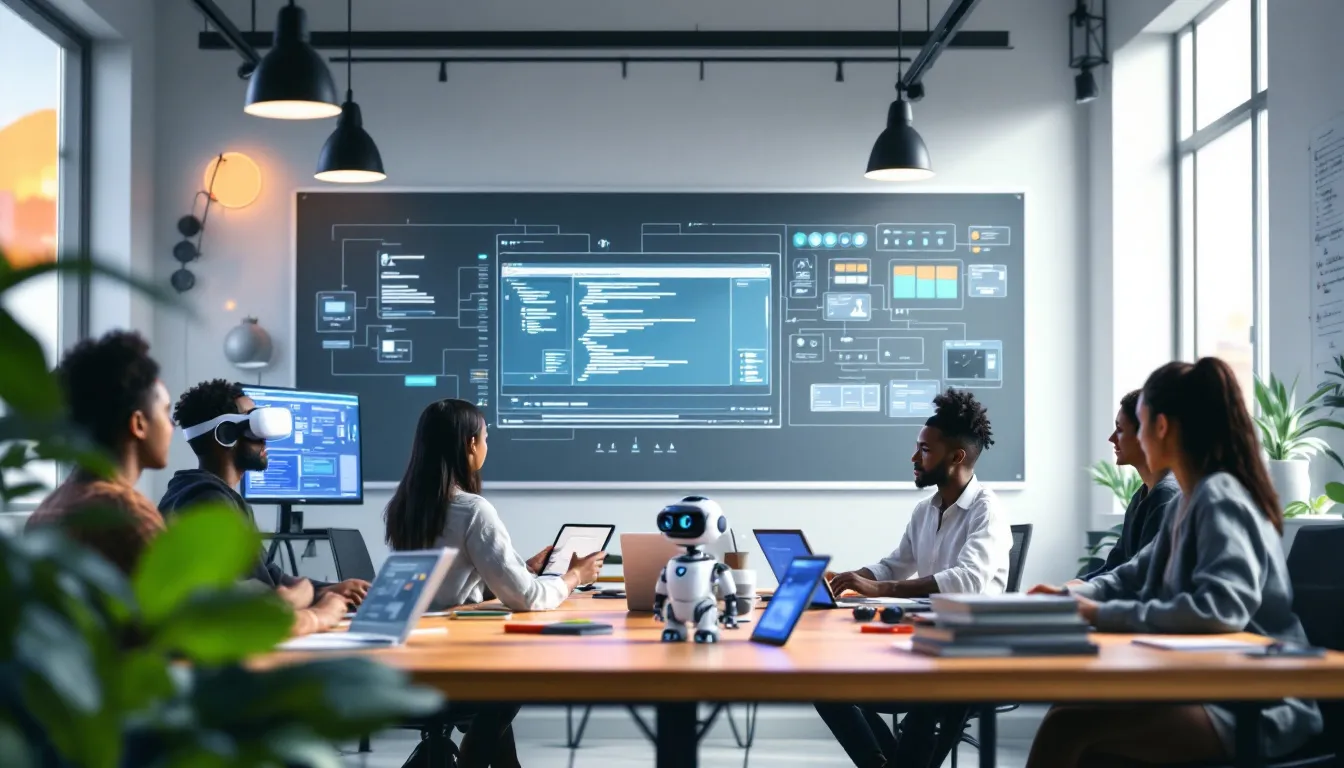
Creating custom AI agents has become more accessible with specialized platforms and frameworks that simplify agent development and deployment. These frameworks provide essential tools, libraries, and integrations, enabling developers and non-developers alike to build agents that can learn, automate tasks, and interact with users effectively.
Best Platforms for Creating Custom AI Agents

1. MosaicML
MosaicML offers a robust platform for training and deploying large language models, allowing users to customize models to their specific requirements. Mosaic’s focus on efficiency and scalability makes it ideal for those looking to train AI agents for high-stakes applications like finance, healthcare, and research.
2. LangChain
LangChain is a popular framework for building language-based AI agents that can perform complex tasks by chaining together multiple prompts and models. With LangChain, developers can create sophisticated, multi-step workflows that integrate with external APIs, making it ideal for chatbots, data processing, and research agents.
3. Auto-GPT
Known for pioneering autonomous AI agents, Auto-GPT is an open-source project that enables the creation of agents capable of completing tasks with minimal human oversight. Auto-GPT agents leverage advanced language models to set goals, make decisions, and learn from actions, making them suitable for real-time applications like financial analysis and customer support automation.
4. Wordware (YC S24)
Wordware (wordware.ai) is an innovative platform that acts as a web-hosted IDE specifically designed for non-technical users collaborating with AI Engineers. Wordware treats prompting as a programming language, moving beyond low/no-code blocks to provide users with granular control over AI agents. It’s ideal for building task-specific AI agents that streamline workflows in marketing, customer service, and personalized engagement.
Problem With Popular Frameworks: Mosaic, LangChain, Auto-GPT

While frameworks like Mosaic, LangChain, and Auto-GPT offer powerful capabilities for building AI agents, they come with significant challenges. One of the main issues is that developing agents within these codebases often demands substantial engineering effort. This complexity can create barriers to collaboration with domain experts, who may not possess the technical skills required to contribute effectively. As a result, valuable insights from non-technical team members may be overlooked, limiting the potential of the AI agents being developed.
Additionally, the heavy reliance on abstractions in these frameworks can obscure the underlying processes, making it difficult for users to understand how their agents operate. This lack of transparency not only hampers debugging and optimization efforts but also inhibits rapid iteration. Teams may find themselves stuck in a cycle of trial and error, as making modifications requires navigating through layers of abstraction. Consequently, while these frameworks provide powerful tools, they can also complicate the agent-building process, ultimately affecting productivity and collaboration.
Using Multiple AI Agents

In complex environments, using multiple AI agents, known as a multi-agent system or multi agent framework, can significantly enhance productivity and problem-solving capabilities. Multi-agent systems involve multiple AI agents, each with a specialized role, working together to achieve a larger objective. This approach is particularly valuable in areas like automation, customer service, and data analysis, where tasks often require different types of expertise.
Benefits of Multi-Agent Systems
1. Scalability
Multi-agent systems scale efficiently, allowing organizations to add or modify agents as tasks evolve. This scalability makes it possible to handle large volumes of data, processes, or requests without overwhelming a single agent.
2. Specialization and Efficiency
Each agent can be assigned specific, specialized tasks—such as data processing, decision-making, or communication—allowing the system to operate more efficiently than a single, general-purpose AI. For example, a customer support system might use separate agents for answering FAQs, handling complaints, and upselling products.
3. Resilience and Flexibility
With multiple agents, the system can be more resilient to errors or unexpected scenarios, as other agents can compensate for or adapt to changes in real time. This flexibility ensures continuity in operations and can provide backup if one agent encounters an issue.
How to Connect and Coordinate Multiple Agents

1. Define Roles and Tasks
To connect multiple agents effectively, it’s essential to assign specific roles and tasks to each agent based on its capabilities. For example, in an e-commerce setting, a multi-agent system might use one agent for product recommendations, another for tracking orders, and a third for processing returns.
2. Enable Communication Protocols
Establish communication channels between agents to enable information sharing. Using protocols like REST APIs or WebSockets, agents can exchange data or status updates, helping them work together and keep each other informed about progress or changes in task priorities.
3. Implement a Central Coordinator
For more complex systems, a central coordinator or orchestration layer can manage agent interactions, ensuring each agent completes its task in sync with the others. This coordinator can prioritize tasks, allocate resources, and resolve conflicts to maintain a streamlined workflow.
4. Monitor and Optimize
Continuously monitor the system to ensure optimal performance. Analyze how agents interact, look for bottlenecks or inefficiencies, and make adjustments as needed. Regular monitoring helps ensure that agents are working effectively together and can adapt to changes in workload or task requirements.
By combining specialized agents in a well-coordinated system, organizations can leverage AI’s full potential, optimizing workflows, and creating robust solutions that respond dynamically to complex challenges.
Building Your Own AI Agent

Creating a custom AI agent involves careful planning, from setting clear objectives to refining the final product. Here’s a step-by-step guide to get you started and tips on choosing the right framework and tools.
Step-by-Step Guide: From Defining Objectives to Testing & Iteration
1. Define Objectives
Start by clearly identifying what you want your AI agent to achieve. Is the goal to automate a specific task, provide personalized recommendations, or assist in data processing? Clear objectives will guide every stage of development and help you choose the right tools and frameworks.
2. Identify Required Capabilities
Decide on the skills and functionalities your agent will need. For instance, if it’s a customer support agent, it may need natural language processing (NLP) and sentiment analysis. A research assistant, on the other hand, might need web scraping and summarization capabilities. List these requirements before selecting any frameworks.
3. Choose a Framework
Choose a development framework that aligns with your goals. Platforms like LangChain and Wordware are versatile for building agents that need customization, while Auto-GPT is ideal for those who want to quickly deploy agents with extensive built-in capabilities. Mosaic and other specialized libraries offer additional customization for more advanced needs.
4. Set Up Integrations
Integrate the necessary tools and APIs your agent will interact with. This could include databases, CRM systems, or external APIs like OpenAI’s for language processing. These integrations are key for your agent to gather, process, and deliver information as needed.
5. Train and Fine-Tune
If your agent relies on machine learning, you may need to train it with relevant data. For NLP-based agents, fine-tuning can improve responses and performance. Testing on a small scale will allow you to refine outputs based on real-world scenarios before full deployment.
6. Test, Monitor, and Iterate
Testing is critical to ensure that your AI agent functions as intended. Run it through various scenarios, gather feedback, and make improvements. Iterative testing helps refine accuracy and functionality, preparing your agent for reliable, ongoing use.
Tips for Choosing the Right Framework and Integrating Necessary Tools

1. Consider Your Technical Requirements
Choose a framework that supports the technical demands of your project. For example, LangChain offers extensive support for NLP tasks, while Wordware’s IDE is ideal for collaborative agent-building with a focus on non-technical domain experts.
2. Look for Scalability
If you anticipate needing more agents or functionality in the future, opt for a framework that supports scalability. Platforms like Mosaic and Wordware make it easier to scale projects, add new agents, or expand current capabilities as your needs grow.
3. Prioritize User-Friendliness
Especially if non-developers are involved in building or managing the agent, choose tools that don’t require extensive coding. Wordware, for example, provides an accessible interface suited to non-technical users collaborating with AI engineers, making it a strong choice for mixed teams. Later on you can deploy an agent via API to ensure seamless integration.
4. Ensure Data Security
Many agents interact with sensitive data. Select tools and frameworks that offer secure data handling and align with industry compliance standards, especially if your agent operates in fields like finance or healthcare.
5. Optimize for Flexibility and Customization
The more flexible the platform, the better you can tailor your agent to meet specific tasks. A customizable framework like LangChain lets you build specialized workflows, while Auto-GPT offers pre-built options for fast deployment with some customization.
By following these steps and carefully choosing tools, you can create an AI agent that not only performs its tasks effectively but also integrates seamlessly with your existing processes, scaling and adapting as your needs evolve.
How do I make my own AI agent?
Let's recap. There are 6 steps of building an AI agent:
- Define Objectives: Determine what tasks you want your agent to accomplish.
- Identify Required Capabilities: Decide on the skills and functionalities your agent will need.
- Choose a Framework/Platform: Select a suitable framework like LangChain or Mosaic based on your needs.
- Set Up Integrations: Incorporate necessary tools for data retrieval, processing, or communication.
- Train and Fine-Tune (optional): If your agent relies on machine learning, you may need to train it with relevant data.
- Test & Iterate: Continuously test your agent's performance and refine its capabilities based on feedback.
AI Agents for Business Efficiency

AI agents are increasingly being used in business to streamline operations, automate workflows, and enhance customer support. These agents can handle routine tasks, analyze data, and improve team coordination, allowing employees to focus on higher-value work.
How can AI be used for business?
AI can significantly enhance business operations through:
- Customer Service Automation: Chatbots can handle inquiries 24/7 without human intervention.
- Data Analysis & Insights Generation: Predictive analytics help businesses make informed decisions based on historical trends.
- Process Automation: Streamlining repetitive tasks such as invoicing or inventory management improves efficiency.
How AI Agents Enhance Workflow Automation and Customer Support
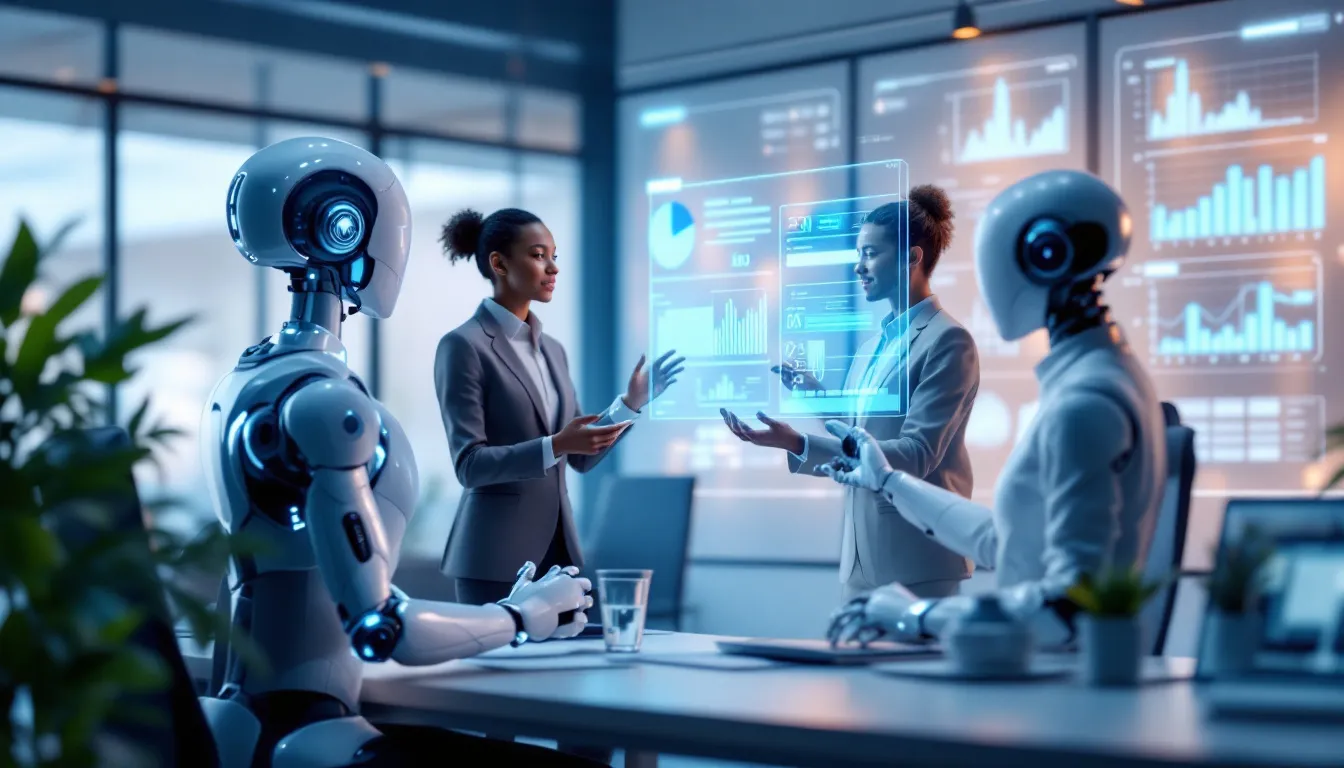
AI agents are ideal for automating repetitive tasks, such as responding to customer inquiries, scheduling meetings, or managing data entry. In customer support, AI-powered chatbots and virtual assistants can handle common questions, freeing up support staff for more complex issues. This automation not only increases efficiency but also provides a faster, consistent customer experience. Additionally, by leveraging NLP and machine learning, agents can offer personalized responses and gain insights from customer interactions, continuously improving over time.
Use Cases in Data Analysis, Process Automation, and Meeting Coordination
Data Analysis

AI agents can sift through large datasets, identify patterns, and generate actionable insights. In finance, for example, they can analyze market trends, provide forecasts, and flag anomalies. In marketing, they can analyze customer data to segment audiences and optimize campaigns. These insights enable more informed decision-making, saving hours of manual analysis.
Process Automation
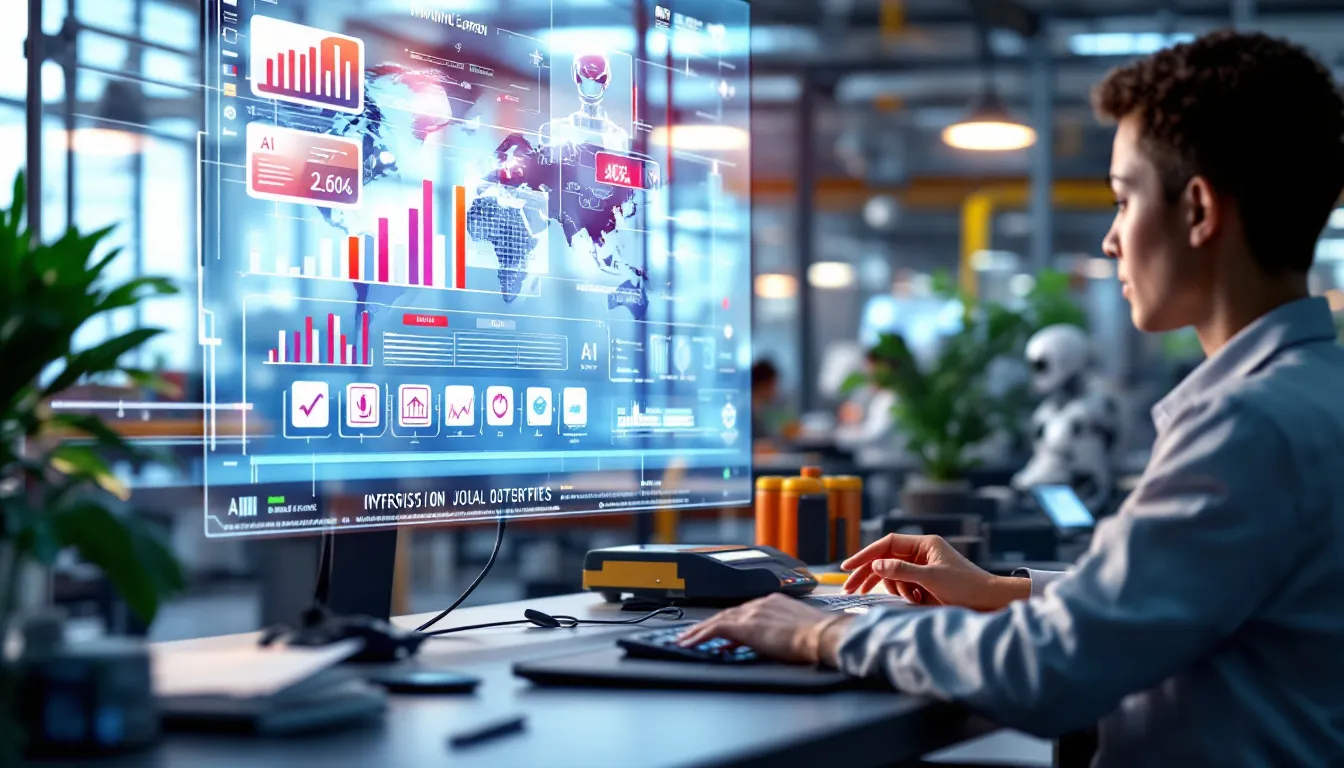
From invoicing and payroll to compliance checks, AI agents automate processes that require repetitive actions, ensuring accuracy and reducing time spent on administrative tasks. In manufacturing, for example, agents can monitor production lines, track supply levels, and even manage quality control checks, improving overall efficiency.
Meeting Coordination
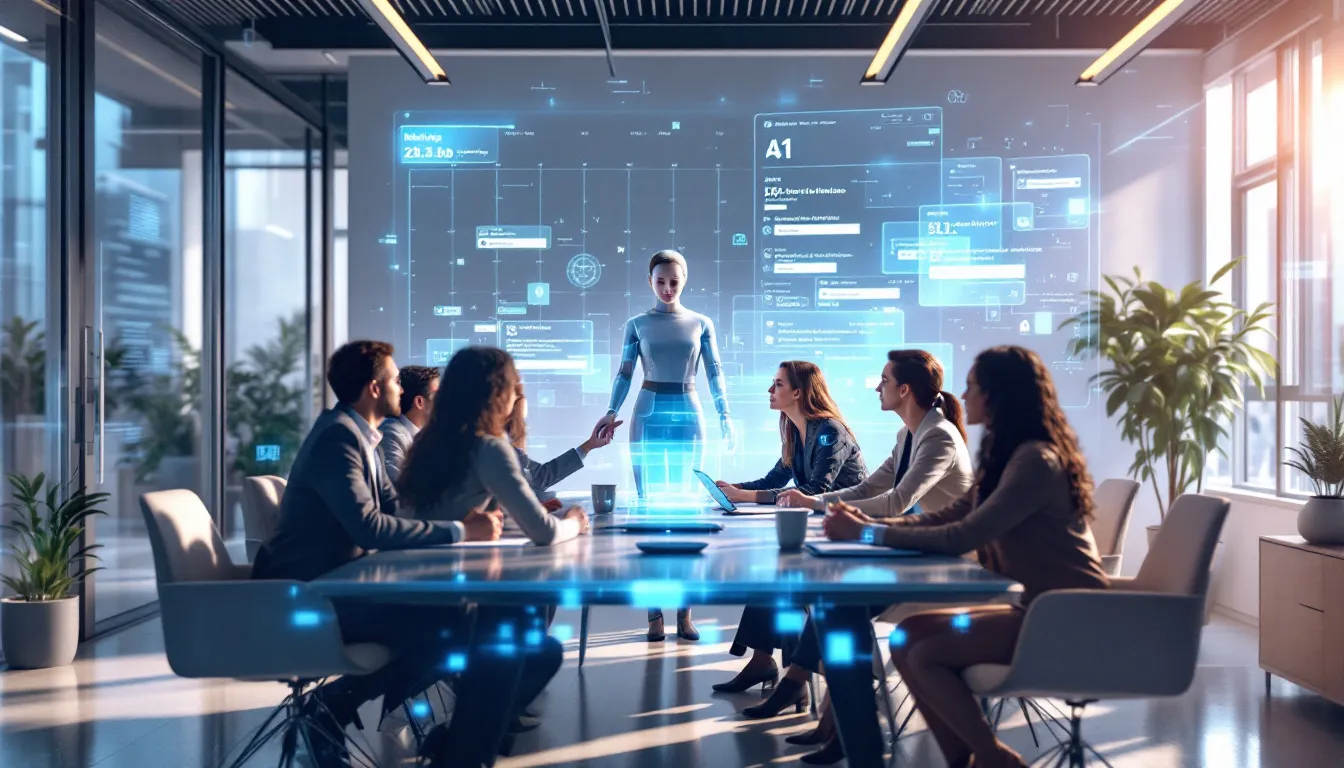
AI agents like scheduling assistants handle meeting coordination, finding optimal times based on team availability, sending calendar invites, and following up with reminders. They can also summarize meeting notes or create action lists, ensuring that key points aren’t missed. This functionality keeps teams organized and reduces the administrative burden associated with meetings.
By implementing AI agents in these areas, businesses can increase productivity, reduce costs, and create smoother workflows, all while freeing their teams to focus on strategic, creative tasks.
In conclusion, as businesses increasingly adopt AI technologies, understanding the capabilities and types of AI agent tools becomes essential for leveraging their full potential. Whether you're looking to automate customer service or enhance operational efficiency, selecting the right tools and frameworks will pave the way for success in an increasingly competitive landscape.
Conclusion
AI agents have emerged as transformative tools, redefining what productivity means in a world where time is a premium commodity. These intelligent assistants are not just gadgets but powerful assets that can automate, streamline, and even personalize how we work and interact, allowing us to focus on the tasks that truly matter. From organizing projects and managing communications to generating code and enhancing creativity, the capabilities of these tools have reached an unprecedented level in 2025, making it a landmark year for AI in our professional and personal lives.
The diversity of available AI agents means there’s something for everyone, regardless of the task or industry. Whether you’re in need of an agent that enhances workflow, supports your creative projects, or assists with data management, there’s an AI tool designed specifically to meet your requirements. This variety allows you to carefully select the tools that align best with your objectives, maximizing the productivity gains these agents offer.
Now, it’s time to take action. Dive into these tools, experiment with their capabilities, and see firsthand the impact they can have on your day-to-day productivity. Embrace the potential of AI agents and unlock new possibilities for efficiency, creativity, and growth in 2025.